A for AGI
Companies like Google and OpenAI are aiming for Artificial General Intelligence or a machine that can do anything the human brain can do. Is it possible? There is disagreement among scientists but will that stop AGI from being a (marketing) goal?
B for Bias
Generative AI can be as good or bad as the literature or paintings the model has studied. So if an AI model is fed data that is, say, more right wing, the outcome would be similar. If a model is fed paintings that are biased towards Cubism, the outcome would show. The problem with AI is bias.
C for Compute
For a machine learning system to evolve, compute is an important factor. What is the amount of processing power required to train AI? When GPT-3 was trained in 2020, it needed 600,000 times more computing power than the one in 2012. This will continue to increase and so will be the cost to deploy cutting-edge AI models. This, in turn, leads to increased carbon emissions.
D for Diffusion Models
By now, many of us have created AI images, like a snow-covered Victoria Memorial. Take the case of DALL-E. The user describes a set of features and DALL-E generates images that include these features. And the bigger image has bits and pieces in the mould of images that already exist. Then a neural network — called a diffusion model — creates the image and comes up with the pixels needed to make the features come to life. The diffusion model is trained on a series of images in which noise is slowly added until there is a sea of random pixels. Then the model learns to recover the data by reversing the process.
E for Emergent Capabilities
Earlier this year, Elon Musk, Apple co-founder Steve Wozniak and others signed a letter that urged “a stepping back from the dangerous race to ever-larger, unpredictable black-box models with emergent capabilities”. Say a model — to do certain things — is released but soon we find it can do something that wasn’t predicted, like hacking networks.
F for Foundation Models
The advanced systems that underpin general purpose AI services, like OpenAI’s ChatGPT and Google’s Bard chatbot. There is something called the Foundation Model Transparency Index that rates large AI language models on how transparent they are.
G for GPT
Generative Pre-trained Transformer or GPT is a neural network that works like a human brain and is trained on input, like massive data sets, to produce outputs or answers to user questions.
H for Hallucination
If you ask something like ChatGPT or Bard a question, the answer will turn up promptly but what if the answer is false? This is called hallucination.
I for Intelligence Explosion
What if an AI model reaches a level after which it begins to train itself certain things? This is what worries us.
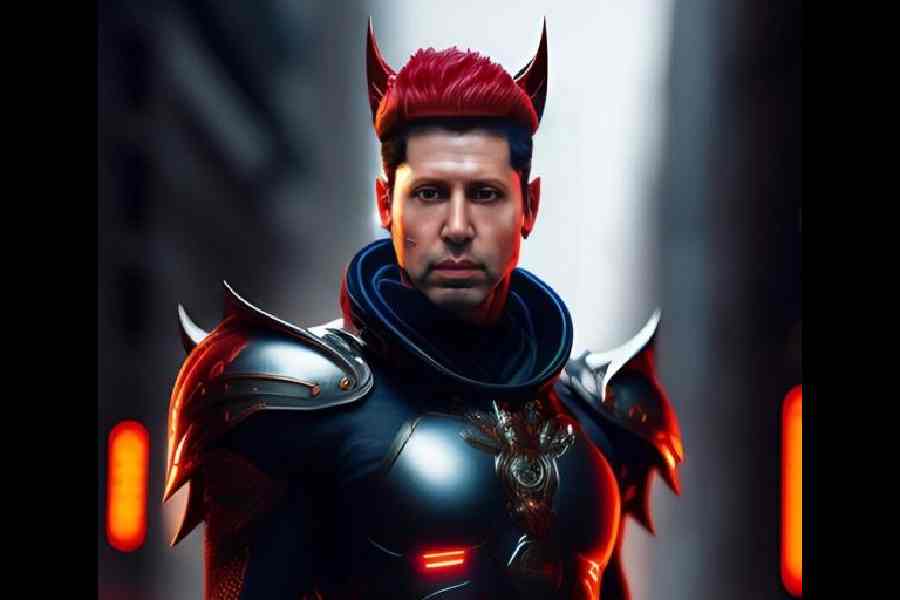
AI-generated image of Sam Altman
J for Job Insecurity
Need I say more?! Every profession is undergoing a change because AI models are becoming more intelligent.
K for Knowledge Graphs
Unstructured data needs to be transformed into knowledge graphs, which users can then query using natural language. A cat and a dog have more connections than a cat and an eagle.
L for Large Language Models
Large language models improve as they are fed more data. Early models appeared around five years ago but today they can even tutor you in a foreign language.
M for Machine Learning
The more data the machine gets access to, the more it learns. The knowledge base is continuing to get smarter and there is far more consistency now than, say, two years ago.
N for Narrow AI
This kind of AI can do a specific task, like a self-driving car driving itself and knowledge gained from performing that task will not automatically be applied to other tasks.
O for Open Sourcing
There is debate about making AI models freely accessible and not keep it restricted to a few powerful tech companies. Open sourcing may lead to dangerous situations but having it tied to a big company can also be problematic.
P for Pattern Recognition
For machine learning to be successful, machines need to learn pattern recognition while going through vast troves of data.
Q for Quantum Machine Learning
Quantum processes meet supercharged machine learning. As a team of Google AI researchers wrote in 2021: “Learning models made on quantum computers may be dramatically more powerful…potentially boasting faster computation [and] better generalisation on less data.”
R for Regulation
In the coming months, we will hear about government regulation of AI around the globe. The world’s first comprehensive laws to regulate AI have already been agreed between the European Parliament and EU member states.
S for Sam Altman
As the co-founder and CEO of OpenAI, Sam Altman has become the poster boy of AI and chatbots.
T for Training Data
More data there is, quicker AI models can learn. And more refined will be the results.
U for Unsupervised Learning
When AI models are given unlabeled data and have to make sense of it without instructions. In simple terms, when machines “teach themselves”.
V for Virtual Assistants
You already know about Google Assistant, Siri, Alexa and the likes. With a new generation of artificial intelligence tech available, these will become more powerful.
W for Worry
Everyone is worried about how AI will reshape our work culture in the next five years and how bad actors can take advantage of flaws in AI systems.
X for X.A.I.
This is explainable AI or when AI can show us how it came to its conclusions.
Y for Yottabyte
It is said that GPT-4 has been trained on one petabyte dataset. But GPT models are getting bigger. So, 1024 petabytes make an exabyte and 1024 exabytes are in a zettabyte and 1,024 zettabytes make a yottabyte. We have the names but now let’s see how quickly the models evolve.
Z for Zero Shot Learning
There are always things that’s out of syllabus, like a cat acting the role of a traffic cop (this is, of course, exaggeration). What if the AI system can’t understand what it is dealing with? It will try to extrapolate from training data to identify something that hasn’t been fed into the system. Or what we call an “educated guess”.
Mathures Paul