A few months ago, OpenAI announced its much-anticipated entry into the search market with SearchGPT, an AI-powered search engine. It accentuates the trend of people looking for a search engine that goes beyond thousands of links, like you find on Google. Wouldn’t it be nice to have a search engine that gives importance to the many Indian languages?
The team of Subhash Sasidharakurup, Dileep Jacob and Vinci Mathews is doing just that with Nofrills AI. You may know them from Kochi-based Watasale, which Amazon acquired a couple of years ago. What are they trying to achieve with Nofrills AI? Here’s what they have to say.
What is Nofrills.ai and why should one use this over Google or Bing?
Nofrills AI is a powerful search engine designed to help users effortlessly discover information, products, and services in their preferred language. It operates on our proprietary model architecture, WISE (Wired Intelligence for Semantic Encoding), which leverages transfer learning with open weights on a revolutionary neuro-symbolic structure. With a simple and intuitive interface, using Nofrills AI is as easy as visiting www.nofrills.ai, selecting your language, and asking questions. Available free of charge to everyone, Our mission is to break down language barriers and make AI-driven progress accessible to all, empowering individuals from diverse backgrounds to explore, learn, and grow.
Traditional search engines, such as Google and Bing, typically provide users with a list of URLs in response to their queries, often prioritising paid and artificially optimised listings. Unfortunately, the URLs that actually contain the answers to the user’s queries are often buried deep within the second or third page of results, leaving users to sift through each URL to determine whether it’s a clickbait or a reliable source of information. This process can be overwhelming, leading users to abandon their search with inaccurate or incomplete information.
While Google and Bing excel at rapidly providing links for keyword-based searches (for example, flight charges, PGs near me), they struggle to provide accurate sources for queries asked in natural language. Nofrills AI is designed to bridge this gap by understanding natural language and providing a concise summary of the information users are seeking and directing them to the exact sources (URLs) containing detailed content, saving them from visiting irrelevant URLs. The short summary from Nofrills’ sources helps users avoid unnecessary detours and land on the exact information they’re looking for.
Further, Google and Bing are unable to comprehend follow-up queries asked by users on a result, treating every query as a new one. For instance, when you retrieve results from Google for a keyword like “hotels near me,” you cannot ask a natural follow-up question like “How many offer free flexible checkout time?” You need to frame a new keyword for that, and even then, it will still provide the same listing (since the indexing is not based on merit but rather on who pays more or spends more money on optimising their pages).
In contrast, Nofrills allows you to ask unlimited follow-up questions in a thread, providing accurate answers and sources in a language you understand.
The key distinction of Nofrills AI from that of Google, Bing, or any other AI-based answer engines is its ability to understand and communicate in any Indic language with the same accuracy as English
What are the advantages Nofrills offer when it comes to AI-driven search in local languages?
Unlike traditional search engines like Google and Bing, which are designed to cater to keyword-based searches, Nofrills AI is revolutionising the way we search for information. For years, people have had to rely on posting questions on platforms like Reddit and Quora to get answers in natural language, which often comes with the risks of biased and inaccurate responses. Moreover, traditional search engines struggle to understand follow-up questions, forcing users to spend precious minutes scouring through multiple URLs to find a reliable source.
The limitations of traditional search engines are even more pronounced when it comes to natural languages. A glance at Google’s search data reveals that the majority of searches are keyword-based and predominantly in English. This is where Nofrills AI comes in — bridging the gap by allowing users to search in natural language and ask follow-up questions in any language they prefer. What’s more, Nofrills AI guides users to reliable sources within seconds.
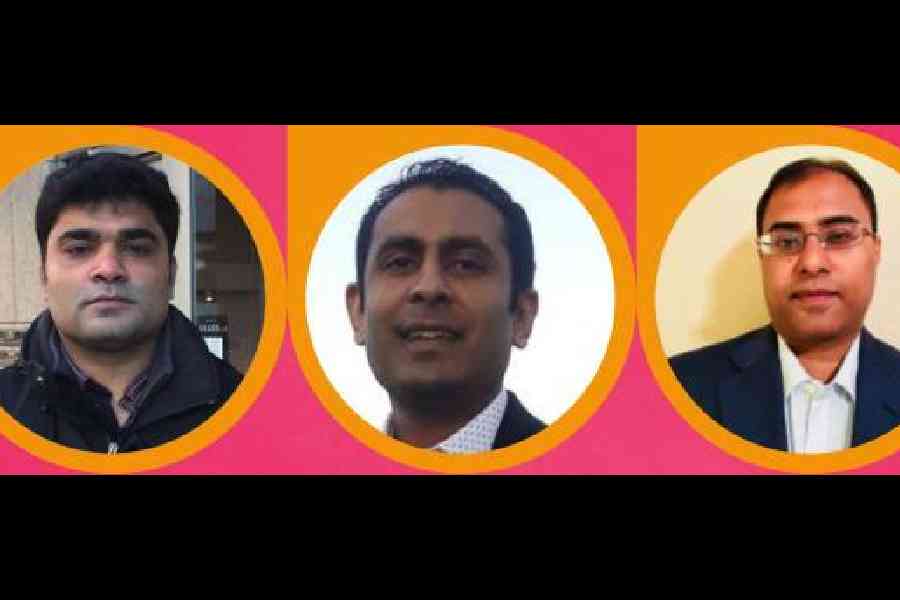
Subhash Sasidharakurup, Dileep Jacob and Vinci Mathews of NoFrills AI
By empowering users to search and communicate in their preferred language, we believe that Nofrills AI will enable them to discover information, products, and services, ultimately benefiting from digital advancements. We’re confident that in the coming years, we’ll change the lives of millions of non-English speaking individuals by opening the doors to the digital realm, making it more inclusive and accessible to all.
What are the challenges in offering local language support in AI-driven search results?
When it comes to interpreting search results or other advanced applications like Retrieval-Augmented Generation (RAG), many models struggle with Indic languages. This challenge stems from a systematic issue: existing tokenisers require approximately 10 times more tokens to convey the same meaning in Indic languages compared to English. This increases both training and inference costs, placing a heavy burden on resources due to the high number of tokens. As a result, models tend to make errors when interacting in Indic languages.
In India, researchers believe that developing an Indic tokeniser will solve this issue. However, I disagree with this approach. The inherent differences between Indic languages and English, such as the larger number of letters and complex grammatical structures, cannot be addressed solely by developing an Indic tokeniser. Indic languages like Hindi, Kannada, and Tamil have intricate inflectional systems, where words change form based on tense, case, number, and gender. They also frequently use suffixes, prefixes, and compound words, leading to longer tokens.
Developing an Indic tokeniser may reduce token counts, but it will struggle to match the token economy of English due to the inherent nature of Indic languages. Or we need to consider overhauling the vector representation of Indic tokens by reducing the number of dimensions to address the increased token count. However, this is a complex task that demands substantial modifications to both the embedding and transformer architectures.
Moreover, training Indic languages requires significantly more resources compared to English, which is a challenge in itself, given the limited investment in training models in English. Further, the amount of digital information available in Indic languages is limited. While local syllabi up to the 10th standard provide some digital data, it remains insufficient.
Using translation tools is another option, but these tools often lack context and nuance, resulting in inappropriate translations.
To address these challenges, we took a different approach from the beginning. We developed a language helper model with enhanced capabilities to assist our core model. Our core model leverages open weights from transfer learning and fine-tuning to enhance reading comprehension. This combination enables our architecture to read, interpret, and communicate effectively in any Indic language.
Digitally low-resource languages (compared to say English, Mandarin or French) like Bengali or Swahili are difficult to work with on search engines like Perplexity or Nofrills. How are you making search in these languages accurate as well as cost effective?
Yes, this is indeed a significant challenge faced by Perplexity and other AI search engines. The availability of digital information in Indic languages is quite limited, which often leads to search engines like Perplexity providing inaccurate answers. This problem extends beyond search engines, affecting models like GPT-4 as well, particularly in the context of retrieval-augmented generation (RAG) for Indic languages.
To address this, we have developed a language helper model with enhanced capabilities designed to support our core model. This combination allows our system to read, interpret information from any language and communicate that information effectively with users in their preferred language.
When it comes to cost, the economics of large language models (LLMs) at scale are largely determined by the number of tokens processed. The higher the token count during training and inference, the higher the cost. Our innovative architecture allows us to maintain costs similar to those of processing English, even when working with Indic languages. For instance, if we were to rely on GPT API and Google Search API, our costs would be at least 10 times higher for answering queries in Indic languages compared to our current expenses. This significant cost advantage sets us apart from others in the industry.
Tell us about your team. Your previous startup, Watasale, was acquired by Amazon. What advantages does Nofrills offer that may align with the objectives of a larger company in the near future?
As founders of a startup acquired by one of the most valuable companies in the world with a trillion-dollar market capital, Subhash Sasidharakurup, Dileep Jacob and Vinci Mathews bring a robust entrepreneurial experience and a deep commitment to innovation, highlighted by patents that reflect our team’s dedication to advancing technologies.
Our collective journey spanning over two decades encompasses a broad spectrum of professional and management roles and is enriched by our international experiences in USA and other regions.
With operations established in the US and India, our diverse expertise converges to propel innovation in AI and related fields. The team comprises experienced professionals from Watasale and Amazon.
What sets us apart is the fact that we have always focused on spending on activities that contribute to the growth of the company.
Why do you call the AI startup Nofrills?
As the phrase ‘no frills’ means “without unnecessary extras, especially ones for decorations,” our product is intended to purely do the task at hand: create a product that is simple, accurate, and easy to use.